The cerebellum is thought to play a critical role in procedural learning, but the relationship between this function and the underlying cellular and synaptic mechanisms remains largely speculative. Synaptic plasticity is known to be distributed and to occur at several sites in the granular layer, molecular layer, and deep cerebellar nuclei. The cerebellum implements a generalized computational algorithm allowing the system “to learn to predict the timing between correlated events”, in a rich set of behavioural contexts. In sensorimotor paradigms, plastic changes over multiple synapses evolve trial-by-trial, regulating the timing of neuronal discharge and fine-tuning high-speed movements on the millisecond timescale. Inter-dependent learning rules are defined matching experimental data, so to predict the emerging behavioural outcomes by healthy or altered cerebellar networks.
A PhD student involved in this research topic will have the opportunity to learn informatics programming and mathematical frameworks in neuroscience, and to deal with plasticity rules.
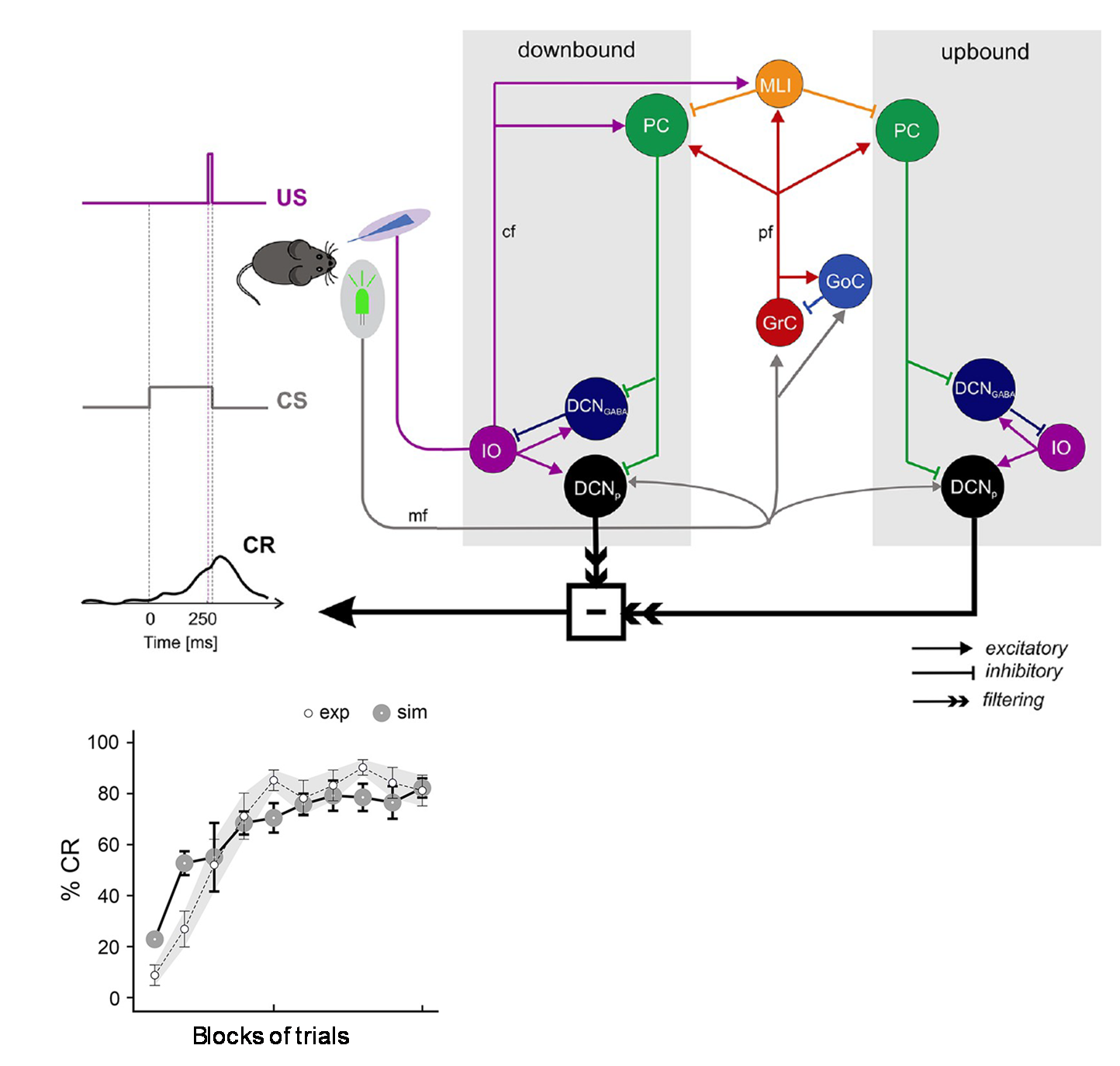
Network model structure and dynamics. Schematics of the olivocerebellar circuit model made of two micromodules including a cerebellar cortical microzone (granule cells – GrC, Golgi cells – GoC, Purkinje cells – PC, Molecular Layer Interneurons – MLI) along with the DCN (deep cerebellar nuclei) and IO (inferior olive). The downbound and upbound microcomplexes differ for their climbing-fiber (cf) input and for neuronal and plastic mechanisms, but share the same mossy fiber input and molecular layer interneurons. The classical eyeblink conditioning task is reproduced: the neutral conditioned stimulus (CS), and the unconditioned stimulus (US) signals are conveyed through the mossy and climbing fiber pathways, respectively, which activate the circuit determining the DCN output activity. The contribution of downbound and upbound DCN neurons is convolved with a gaussian filter and algebraically summed to generate the behavioural output: trial-by-trial the conditioned response (CR) is learned through plasticity mechanism and anticipates US when DCN activity crosses a behavioural threshold.
References
- Geminiani A, Casellato C, Boele H-J, Pedrocchi, De Zeeuw C I, D’Angelo E. Mesoscale simulations predict the role of synergistic cerebellar plasticity during classical eyeblink conditioning. PLOS COMP BIO. 2024; doi: 10.1371/journal.pcbi.1011277
- Fruzzetti L, Kalidindi HT, Antonietti A, Alessandro C, Geminiani A, Casellato C, Falotico E, D’Angelo E. Dual STDP processes at Purkinje cells contribute to distinct improvements in accuracy and speed of saccadic eye movements. PLOS COMPUT BIO. 2022; doi: 1371/journal.pcbi.1010564
- D‘Angelo E, Mapelli L, Casellato C, Garrido J.A, Luque N, Monaco J, Prestoria F, Pedrocchi A, Ros E. Distributed circuit plasticity: new clues for the cerebellar mechanisms of learning. THE CEREBELLUM 2016; 15(2):139-151; doi: 10.1007/s12311-015-0711-7
- Monaco J, Casellato C, Koch G, D’Angelo E. Cerebellar theta burst stimulation dissociates memory components in eyeblink classical conditioning. EUR J NEUROSCI. 2014; 40(9):3363-3370; doi: 10.1111/ejn.12700